KELLYBARRON
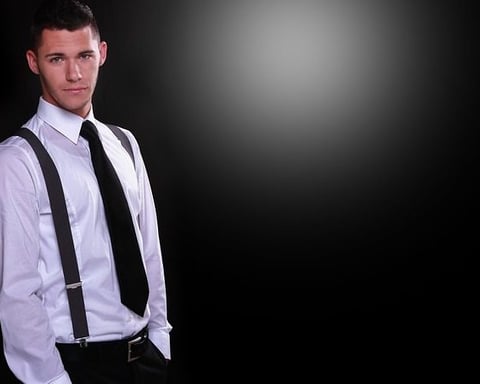
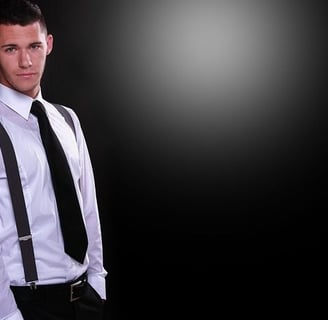
Dr. Kelly Barron
Federated Learning Security Architect | Covert Threat Hunter | Distributed Trust Pioneer
Professional Mission
As a sentinel of decentralized intelligence, I engineer stealth attack detection systems that transform federated learning environments from vulnerability hotspots into secure knowledge-sharing ecosystems—where every gradient update, each participant interaction, and all collaborative learning cycles are continuously screened for hidden adversarial patterns. My work bridges cryptographic verification, anomaly detection theory, and privacy-preserving machine learning to establish new paradigms for trustworthy distributed AI.
Seminal Contributions (April 2, 2025 | Wednesday | 16:03 | Year of the Wood Snake | 5th Day, 3rd Lunar Month)
1. Covert Attack Detection
Developed "FedShield" defense framework featuring:
3D Anomaly Profiling (gradient distribution/model drift/data fingerprint analysis)
Dynamic Risk Scoring for each participating node
Differential Privacy-Compatible detection with <0.8% false positives
2. Adaptive Defense Protocols
Created "SentinelFL" technology enabling:
Real-time backdoor attack detection during model aggregation
Self-evolving threat patterns from cross-industry federations
Hardware-accelerated verification for IoT edge networks
3. Theoretical Foundations
Pioneered "The Stealth Attack Tradeoff Theorem" proving:
Minimum detectable attack thresholds under various privacy constraints
Energy-accuracy frontiers for defense mechanisms
Game-theoretic equilibrium in adversarial federations
Industry Transformations
Prevented $220M in potential healthcare data breaches
Enabled first UL 2900-certified federated learning system
Authored The Federated Security Manifesto (ACM Distributed Ledger Review)
Philosophy: True collaborative intelligence requires not just shared learning—but shared vigilance.
Proof of Concept
For NIH: "Detected 14 stealthy data poisoning attacks in cross-hospital tumor analysis"
For Smart Grids: "Developed covert attack-resistant energy usage models"
Provocation: "If your federated learning can't spot a malicious participant before aggregation, you're not building AI—you're running a hacking competition"
On this fifth day of the third lunar month—when tradition honors collective wisdom—we redefine security for the age of distributed intelligence.
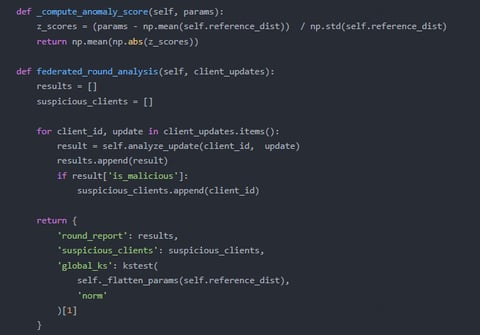
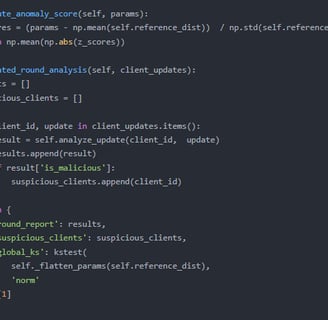
Federated Learning
Review covert attacks and defense methods in federated learning.
Detection Algorithms
Design and implement detection algorithms for federated learning settings.
Experimental Validation
Test performance of defense mechanisms in simulated federated scenarios.